Production Checklist for Web Apps on Kubernetes
Posted: | More posts about kubernetes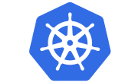
Running applications in production can be tricky. This post proposes an opinionated checklist for going to production with a web service (i.e. application exposing HTTP API) on Kubernetes.
This checklist was shaped by my own limited experience in Zalando, so please see it only as template for your own checklist adopted to your environment. Some items can be considered optional or aspirational, depending on context.
General
Application's name, description, purpose, and owning team is clearly documented (e.g. in a central application registry or wiki)
Application's criticality level was defined (e.g. "tier 1" if the app is highly critical for the business)
Development team has sufficient knowledge/experience with the technology stack
Responsible 24/7 on-call team is identified and informed
Go-Live plan exists incl. steps for potential rollback
Application
Application's code repository (git) has clear instructions on how to develop, how to configure, and how to contribute changes (important for emergency fixes)
Code dependencies are pinned (i.e. hotfix changes do not accidentally pull in new libraries)
All relevant code is instrumented with OpenTracing or OpenTelemetry
OpenTracing/OpenTelemetry semantic conventions are followed (incl. additional company conventions)
All outgoing HTTP calls have a defined timeout
HTTP connection pools are configured with sane values according to expected traffic
Thread pools and/or non-blocking async code is correctly implemented/configured
Database connection pools are sized correctly
Retries and retry policies (e.g. backoff with jitter) are implemented for dependent services
Circuit breakers are implemented
Fallbacks for circuit breakers are defined according to business requirements
Load shedding / rate limiting mechanisms are implemented (could be part of provided infrastructure)
Application metrics are exposed for collection (e.g. to be scraped by Prometheus)
Application logs go to stdout/stderr
Application logs follow good practices (e.g. structured logging, meaningful messages), log levels are clearly defined, and debug logging is disabled for production by default (with option to turn on)
Application container crashes on fatal errors (i.e. it does not enter some unrecoverable state or deadlock)
Application design/code was reviewed by a senior/principal engineer
Security & Compliance
Application can run as unprivileged user (non-root)
Application does not require a writable container filesystem (i.e. can be mounted read-only)
HTTP requests are authenticated and authorized (e.g. using OAuth)
Mechanisms to mitigate Denial Of Service (DOS) attacks are in place (e.g. ingress rate limiting, WAF)
A security audit was conducted
Automated vulnerability checks for code / dependencies are in place
Processed data is understood, classified (e.g. PII), and documented
Threat model was created and risks are documented
Other applicable organizational rules and compliance standards are followed
CI/CD
Automated code linting is run on every change
Automated tests are part of the delivery pipeline
No manual operations are needed for production deployments
All relevant team members can deploy and rollback
Production deployments have smoke tests and optionally automatic rollbacks
Lead time from code commit to production is fast (e.g. 15 minutes or less including test runs)
Kubernetes
Development team is trained in Kubernetes topics and knows relevant concepts
Kubernetes manifests use the latest API version (e.g.
apps/v1
for Deployment)Container runs as non-root and uses a read-only filesystem
A proper Readiness Probe was defined, see blog post about Readiness/Liveness Probes
No Liveness Probe is used, or there is a clear rationale to use a Liveness Probe, see blog post about Readiness/Liveness Probes
Kubernetes deployment has at least two replicas
A Pod Disruption Budget was defined (or is automatically created, e.g. by pdb-controller)
Horizontal autoscaling (HPA) is configured if adequate
Memory and CPU requests are set according to performance/load tests
Memory limit equals memory requests (to avoid memory overcommit)
CPU limits are not set or impact of CPU throttling is well understood
Application is correctly configured for the container environment (e.g. JVM heap, single-threaded runtimes, runtimes not container-aware)
Single application process runs per container
Application can handle graceful shutdown and rolling updates without disruptions, see this blog post
Pod Lifecycle Hook (e.g. "sleep 20" in
preStop
) is used if the application does not handle graceful terminationAll required Pod labels are set (e.g. Zalando uses "application", "component", "environment")
Application is set up for high availability: pods are spread across failure domains (AZs, default behavior for cross-AZ clusters) and/or application is deployed to multiple clusters
Kubernetes Service uses the right label selector for pods (e.g. not only matches the "application" label, but also "component" and "environment" for future extensibility)
There are no anti-affinity rules defined, unless really required (pods are spread across failure domains by default)
Optional: Tolerations are used as needed (e.g. to bind pods to a specific node pool)
See also this curated checklist of Kubernetes production best practices.
Monitoring
Metrics for The Four Golden Signals are collected
Application metrics are collected (e.g. via Prometheus scraping)
Backing data store (e.g. PostgreSQL database) is monitored
SLOs are defined
Monitoring dashboards (e.g. Grafana) exist (could be automatically set up)
Alerting rules are defined based on impact, not potential causes
Testing
Breaking points were tested (system/chaos test)
Load test was performed which reflects the expected traffic pattern
Backup and restore of the data store (e.g. PostgreSQL database) was tested
24/7 On-Call
All relevant 24/7 personnel is informed about the go-live (e.g. other teams, SREs, or other roles like incident commanders)
24/7 on-call team has sufficient knowledge about the application and business context
24/7 on-call team has necessary production access (e.g. kubectl, kube-web-view, application logs)
24/7 on-call team has expertise to troubleshoot production issues with the tech stack (e.g. JVM)
24/7 on-call team is trained and confident to perform standard operations (scale up, rollback, ..)
Runbooks are defined for application-specific incident handling
Runbooks for overload scenarios have pre-approved business decisions (e.g. what customer feature to disable to reduce load)
Monitoring alerts to page the 24/7 on-call team are set up
Automatic escalation rules are in place (e.g. page next level after 10 minutes without acknowledgement)
Process for conducting postmortems and disseminating incident learnings exists
Regular application/operational reviews are conducted (e.g. looking at SLO breaches)
Anything missing on this list? Do you disagree with something? Ping me on Twitter!